Acknowledging that the agent’s learning objective (e.g., maximize immediate reward or sum of reward over the long-term) may or may not line up with performance on the trainer’s intended task (e.g., follow the human trainer), we examined the relationships between reward positivity, temporal discounting, episodicity, and task performance. Contributions of this work include
- empirical support for and justification of the newly noted myopic approach used across all previous projects for learning from human reward;
- the first successful instance of non-myopic learning from human reward and evidence that non-myopic approaches will enhance the effectiveness of teaching by human reward, shifting the burden from users to the robot; and
- providing evidence for the incompatibility of non-myopic learning and episodic tasks for a large class of domains, and conversely providing an endorsement of framing tasks as continuing when learning from human reward.
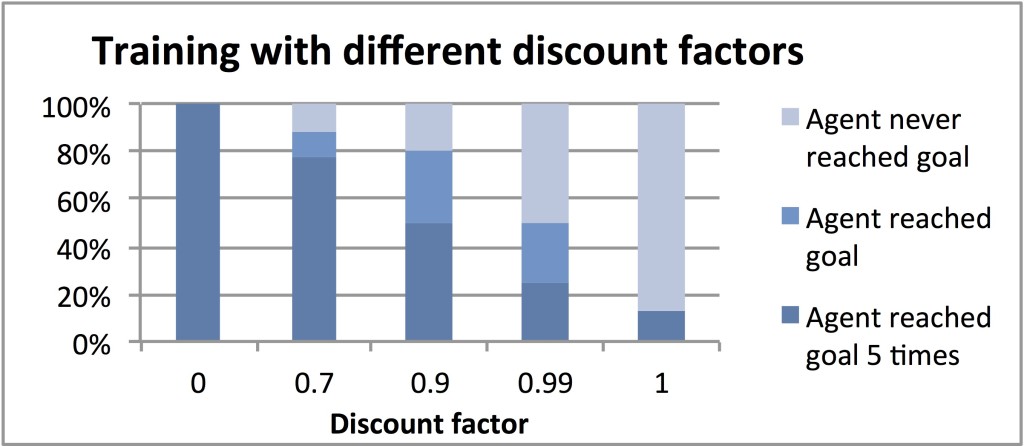
Relevant publications
W. Bradley Knox and Peter Stone. Framing Reinforcement Learning from Human Reward: Reward Positivity, Temporal Discounting, Episodicity, and Performance. Artificial Intelligence, 225, 24–50. August 2015.
pdf (author’s pre-print)
Artificial Intelligence Journal page
The two publications below are subsumed by the AIJ article above.
W. Bradley Knox and Peter Stone. Learning Non-Myopically from Human-Generated Reward. In Proceedings of the International Conference on Intelligent User Interfaces (IUI). March 2013.
[pdf] (2.1 MB)
IUI 2013
W. Bradley Knox and Peter Stone. Reinforcement Learning from Human Reward: Discounting in Episodic Tasks. In Proceedings of the 21st IEEE International Symposium on Robot and Human Interactive Communication (Ro-Man). September 2012.
Finalist for CoTeSys Cognitive Robotics Best Paper award
[pdf] (2.1 MB)
Ro-Man 2012